Start improving with Life QI today
Full access to all Life QI features and a support team excited to help you. Quality improvement has never been easier.
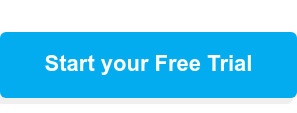
Organisation already using Life QI?
Sign-up
Designing your measurement plan is often one of the most challenging parts of any improvement work. This is true for a single project, and even more so when working on large cross-team or multi organisation collaborative efforts. One of the challenges can be in overcoming ‘data-overload’, which can be a source of wasted time and effort and potentially distract from the purpose of the work, especially when rapid cycle of change and analysis of results is needed. One of the best mechanisms in avoiding data over-load is data sampling.
Sampling is a common approach to simplifying data collection and analysis. It is a process of picking out a few individuals from a larger group that will be representative of the whole.
One of the most commonly encountered examples of sampling is in customer satisfaction surveys. Let’s take an example of a Broadband supplier, or Internet Service Provider (ISP). One of the biggest differentiators in ISPs is the service levels provided, which is generally tracked by surveying customers to identify how many are satisfied or dissatisfied with the service received. Now, an ISP likely has hundreds of thousands of customers – in statistics this is called the ‘population’ (i.e. the entire collection of people, things, items of cases that are being dealt with). It would be very time consuming, expensive and generally impractical to survey every customer to understand customer satisfaction, so to avoid this they use a sample.
In statistics, the sample is just a specific collection of the people, things, items of cases that are drawn from a population in which you are interested. In the case of the ISP, their sample may be 1,000 customers. After surveying their sample of customers, they can calculate what % are satisfied, then apply this to the entire population. Simple.
The biggest benefit of sampling data is that it is much faster and required less effort to collect data for a sample than for an entire population, whilst also allowing you to draw the same conclusions from the data.
Unlike a research study, or customer satisfaction survey, Improvement work is often very time and resource limited. This means that effective use of sampling is even more important in making best use of that. Further to that, the iterative nature of improvement work using methods such as PDSA make rapid data collection and analysis essential.
One of the biggest downsides to sampling is the potential for bias in your dataset. Since you are not collecting data for the whole population, you need to be sure that the sample you chose is as close a representation to the population as possible. If that is not the case, then this leads to bias in your dataset and increases the likelihood of misleading data and errors in your conclusions.
Bias comes in many forms, and the potential for bias will be different depending on the dataset you are working with. Thinking of our ISP example – perhaps they only surveyed customers who had been with them for over 2 years. This could result in a bias known as ‘survivorship bias’, whereby those customers who have been loyal for 2 years are represented, but any customers who left before making it to 2 years are not represented. In QI, survivorship bias is prevalent when researching past QI projects – write-ups on successful projects are plentiful, but those on unsuccessful QI projects rarely get that far.
Another common bias is undercoverage bias whereby some portions of the population are underrepresented in sample. The best approach to minimise bias in your data is to use a recognised sampling technique…
To minimise bias in a sample, its best to select the sample in a systematic way to ensure samples are truly representative of your population. Whist this sounds like an extra level of complexity, in practice, sampling can be simple. The best approach is to use one of the following random sampling techniques….
This is the most common and straightforward sampling techniques and certainly one of the best. In this sample, every individual in a population has an equal chance of inclusion in the sample.
How it works: Each individual is assigned a number, then numbers are drawn at random to select the sample. This could be ‘drawing numbers from a hat’, or tools like Excel can be used to randomly generate the numbers.
Advantages: Provides a truly random sample, its simple to apply on small datasets
Disadvantages: You need a list of every member of the population from the start, it can be cumbersome with large datasets.
Best for: relatively small datasets as the process of selecting individuals becomes cumbersome with a large dataset.
This is a slightly more structured approach than random sampling, making it easier to apply on larger datasets.
How it works: Individuals are selected according to an interval between them in a list. E.g. our ISP may have a list of their customers and decide to sample every 200th customer, so would go down the list and selected customer 200, 400, 600 and so on.
Advantages: Gives a good spread across the population, Easier to apply than simple random sampling, particularly for large datasets.
Disadvantages: You need a list of every member of the population from the start, need to know sample size and calculate sample interval.
Best for: large datasets where a truly random sample is needed.
This is slightly different from the first two approaches and involves first breaking down the population by simple characteristics.
How it works: The population is first divided into groups called ‘strata’ – these strata are defined by characteristics they share (e.g. age, location, ethnic group). Simple random sampling is then used to select individuals from the strata groups.
Advantages: The main advantage this method has over others is the ability to select more from one group over another. This can be proportionate (i.e. if the strata contans 20% of the population, then 20% of the individuals come from that sample), or disproportionate (i.e. the number of individuals selected from a strata is not related to the strata size). Proportionate is the most common, however disproportionate can be useful where some groups are likely to be underrepresented and need stringer representation.
Disadvantages: You need a list of every member of the population from the start, need to know sample size and calculate sample interval.
Best for: datasets that can easily be divided into mutually exclusive sub-groups, particularly if you believe they will take on different mean values for a variable being studied. In the ISP example, they could split the population into strata by geographical location, then select the sample to be representative of each location. This would ensure that all geographical locations are represented proportionally.
This technique also involves splitting the population into groups, known as ‘clusters’
How it works: The population is divided into clusters, then one or more clusters are selected to make up the samples. Unlike ‘stratified random sampling’, in this case all individuals from the chosen clusters are selected for the sample, and no-units from non-selected clusters are used.
Advantages: can significantly reduce admin time and costs
Disadvantages: higher sampling errors than the other random sampling techniques can result in less accurate results.
Best for: situations where speed and convenience are key and accuracy of results is less of a priority.
Sometimes representative (random sampling) is not practical in the timeframe of an improvement project, and in those cases non-representative samples may be used. The main disadvantage of non-representative samples is that they are not random, and as such are not a true representation of your population. These have higher degrees of error, data bias, and inaccurate conclusions.
As the name suggests, this is a sample selected for a specific purpose. It can be used when it seems sensible to focus on a particular group. In our ISP example, they are looking for a broad view of customer opinions so this sampling would not make sense. However, if they wanted to get a view of a new support feature, then it might make sense to canvas only the opinions of the people who have used the feature, or have been using it the longest.
This is really an unstructured selection, and is essentially a case of using what you can access. In the ISP example, they could have just asked those people who happened to call the customer support helpline on a given day. The main problem with this type of sample is that it is not random and has high likelihood of bias.
A sample is only useful if it is representative of the total population, therefore its size will be relative to the total size of the population. In reality, the size of a sample in an improvement project may be constrained by what data are practical to collect.
Its important to appreciate that the size of your sample compared to your total population will impact the confidence in any interpretations of your sampled data. Considering our ISP example, if they have 100,000 customers and sampled only 1,000 (1%) of them, they are going to have a much lower confidence in the results, compared to if they had sampled 20,000 customers (20%).
So what is the right size for a sample? Well, the reality in improvement work is that samples may be determined by what data are practical to collect rather than any calculated number. However, if you are sampling data and want to understand what size the sample should be, then you can use a sample size calculator to determine the optimum size of your sample.
Where time is limited in improvement work, its useful to recognise that the size of the sample is not always the biggest concern and it may be better to focus on ensuring the data you do have is representative of the population. In the article “Sampling considerations for health care improvement”, the authors recommend that for PDSA cycles, teams focus less on the size of the sample, and more on ensureing data are collected consistently on a frequent basis (e.g. daily, weekly, monthly).
Life QI can be used to plot sampled data on Run Charts and Control Charts, just as it can for complete datasets. In some cases, the size of the sample will impact how quickly a change can be identified in the data
When working with sampled data it is a good idea to make that clear in the ‘Operational definition’ of your measure – this is where you can outline the finer details of your measure, including the sampling methodology. Further to this, the ‘Data collection plan’ in Life QI provides the opportunity to make it clear how data are being collected and ensure that data collection is consistent over time. This is of particular importance where the person collecting the data is liable to change, or where multiple teams are involved in collecting data across an improvement collaborative.
HQIP. 2018. An introduction to analysing quality improvement & assurance data.
Bhandari, P. (2023, March 17). Sampling Bias and How to Avoid It | Types & Examples. Scribbr. Retrieved 29 November 2023, from https://www.scribbr.co.uk/bias-in-research/sampling-bias-explained/
SamplingConsiderationsforHealthCare Improvement
https://pubmed.ncbi.nlm.nih.gov/25260103/
Full access to all Life QI features and a support team excited to help you. Quality improvement has never been easier.
Organisation already using Life QI?
Sign-up